Title: Trajectory Privacy Protection with Geospatial AI
Abstract: The prevalence of Location-Based Services (LBS) has led to the generation of large amounts of individual-level trajectory data, which offers opportunities to study human mobility patterns, human-environment interactions, disaster responses, and public health issues. However, trajectory big data also pose significant challenges related to geoprivacy protection and broader social and ethical implications. In this talk, we will discuss three main challenges in trajectory privacy protection, namely the trade-off between privacy and utility, data sparsity and imbalance issues, and endogenous privacy risks in centralized structures. We will also introduce some of our recent work to demonstrate the potential of Geospatial AI in addressing these challenges in trajectory privacy protection.
Bio: Dr. Jinmeng Rao is an AI researcher at Google DeepMind. He was an AI researcher at Google[X]. He got his MS in Computer Sciences and PhD in Geography at UW-Madison supervised by Prof. Song Gao. He holds a M.S. and B.S. in Cartography and GIS from Wuhan University. His main research interest is Geospatial AI and geoprivacy. His articles have been published in top journals and conferences, including IJGIS, TGIS, GIScience, ACM SIGSPATIAL, ACM SIGIR, AAAI, etc. He serves as a reviewer or for the program committee for various journals and conferences, including IJGIS, Annals of GIS, TGIS, JAG, Computers & Geosciences, NeurIPS, ACL, etc. He received the 2024 AAG William L. Garrison Award for Best Dissertation in Computational Geography.
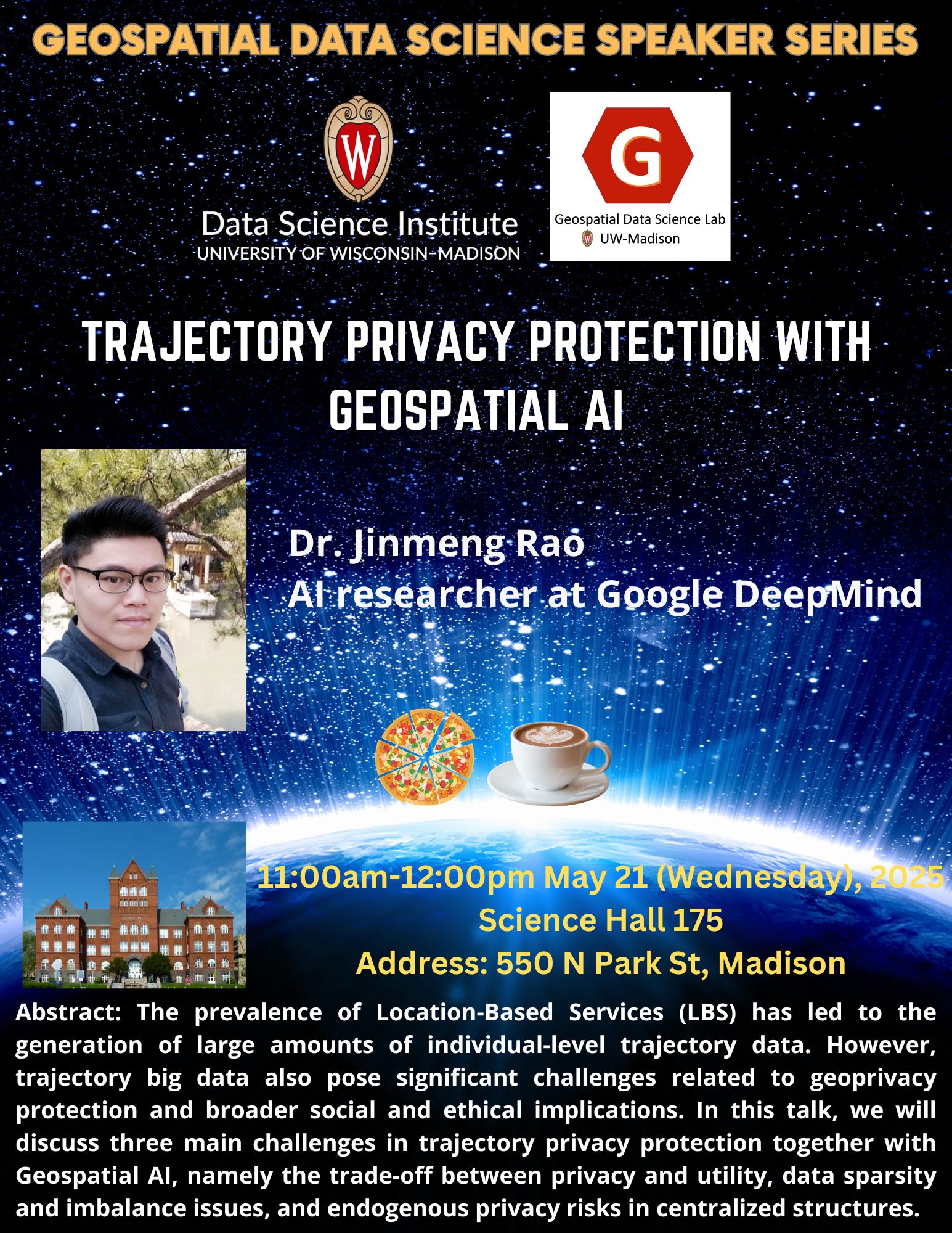