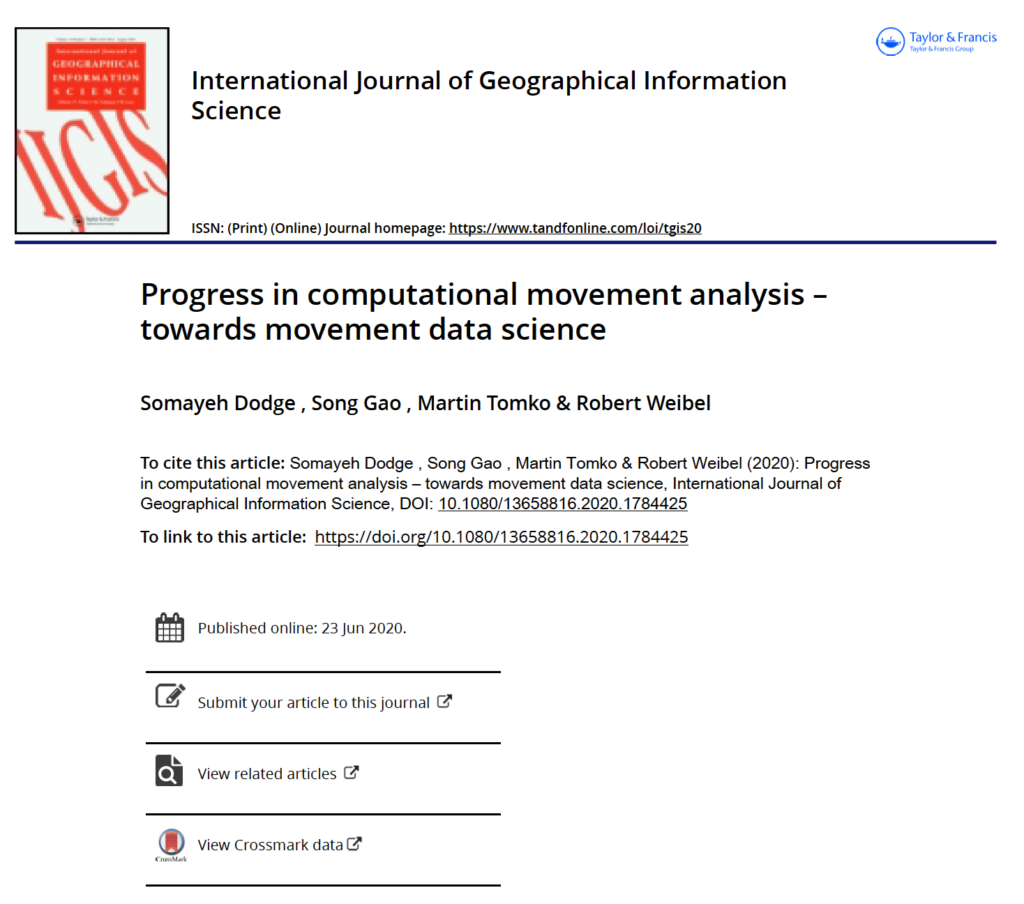
There has not been a time in the history of GIScience when movement analytics and mobility insights have played such an important role in policymaking as in today’s global responses to the COVID-19 crisis. This special section further builds on previous efforts by the editorial team and others from the GIScience community and beyond to advance the body of knowledge in Computational Movement Analysis (CMA). CMA generally refers to series methods and analytical approaches to process, structure, visualize and analyze tracking data and movement patterns to facilitate knowledge discovery and modeling of movement. Specifically, this special section was proposed as part of a pre-conference workshop on Analysis of Movement Data (AMD 2018) at the GIScience 2018 meeting, 28 August 2018, Melbourne, Australia. The focus of this special section is on three aspects of CMA: (1) representation and modeling of movement; (2) urban mobility analytics; and (3) movement analytics using social media data. With the papers presented in the special section, we highlight recent advancements in CMA with the development of methods and techniques for big movement data analytics and utilization of trajectories constructed using user-generated crowdsourced contents such as geo-tagged social media posts. Traditional CMA methods were often developed and evaluated using a smaller set of movement data involving smaller numbers of individuals and contextual variables.
As the momentum to generate more geo-enriched movement data at large volumes, high frequencies and for longer durations continues, this is a timely and significant achievement towards movement data science. As the papers of this special section illustrate, movement data science leverages the advancements in big data analytics, cyberinfrastructure, parallel computing and data fusion to enhance the analysis of large, multi-faceted and multi-sourced movement data. Below are the editorial and the six original papers presented in this special section on the International Journal of Geographical Information Science (IJGIS).
Dodge, S., Gao, S., Tomko, M., & Weibel, R. (2020). Progress in computational movement analysis – towards movement data science. International Journal of Geographical Information Science, 1-6.
Buchin, M., Kilgus, B., & Kölzsch, A. (2019). Group diagrams for representing trajectories. International Journal of Geographical Information Science, 1-33.
Graser, A., Widhalm, P., & Dragaschnig, M. (2020). The M³ massive movement model: a distributed incrementally updatable solution for big movement data exploration. International Journal of Geographical Information Science, 1-24.
Qiang, Y., & Xu, J. (2019). Empirical assessment of road network resilience in natural hazards using crowdsourced traffic data. International Journal of Geographical Information Science, 1-17.
Li, W., Wang, S., Zhang, X., Jia, Q., & Tian, Y. (2020). Understanding intra-urban human mobility through an exploratory spatiotemporal analysis of bike-sharing trajectories. International Journal of Geographical Information Science, 1-24.
Ma, D., Osaragi, T., Oki, T., & Jiang, B. (2020). Exploring the heterogeneity of human urban movements using geo-tagged tweets. International Journal of Geographical Information Science, 1-22.
Xin, Y., & MacEachren, A. M. (2020). Characterizing traveling fans: a workflow for event-oriented travel pattern analysis using Twitter data. International Journal of Geographical Information Science, 1-20.
Moving forward, we see a clear need for more reproducible research in CMA, following a growing mega-trend in data-driven sciences. Data quality and privacy challenges as well as uncertainty in data, analytics, and modeling have been largely overlooked in the CMA literature so far. For a more responsible movement data science, careful considerations should be given to the quality, uncertainty and representativeness of ‘large’ mobility data that are being used for generating important mobility insights for policymaking. Lastly, with the recent exciting developments in data access, as a community, we should think about leveraging this advantage to make movement data science more relevant to real-world problems for the mitigation of societal and environmental challenges such as disease outbreaks, population mobility, natural hazards and human-wildlife conflicts.
Link: GIScience 2020 Workshop on Advancing Movement Data Science (AMD’20) turns to 2021