Title: Spatial Representation Learning: What, How, and Why
Abstract: Spatial representation learning (SRL) aims at learning general-purpose neural network representations from various types of spatial data (e.g., points, polylines, polygons, networks, images, etc.) in their native formats. Learning good spatial representations is a fundamental problem for various downstream applications such as species distribution modeling, weather forecasting, trajectory generation, geographic question answering, etc. In this presentation, we will discuss several recent works from UT SEAI Lab about spatial representation, including various location encoding models (Space2Vec and Sphere2Vec), an SRL deep learning framework (TorchSpatial), and a SRL-powered geo-foundation model (GAIR). We will discuss 1) WHAT is location representation learning? 2) HOW to develop location representation learning models? and 3) WHY do we need them?
Bio: Dr. Gengchen Mai is currently a Tenure-Track Assistant Professor at the Department of Geography and the Environment, University of Texas at Austin. He got his Ph.D. in GIScience from UCSB Geography. Before becoming a faculty, he was a Postdoc at Stanford Computer Science. Before joining UT, he was an Assistant Professor at the University of Georgia. Dr. Mai’s research is Spatially Explicit Artificial Intelligence, Geo-Foundation Models, Geographic Knowledge Graphs, etc. Dr. Mai’s work has been published not only in many top geography/GIScience/Remote Sensing journals but also in many ML/AI conferences such as NeurIPS, ICML, ICLR, ACM SIGIR, ACM SIGSPATIAL, etc. He is the recipient of many prestigious awards including AAG 2021 Dissertation Research Grants, AAG 2022 William L. Garrison Award for Best Dissertation in Computational Geography, AAG 2023 J. Warren Nystrom Dissertation Award, Top 10 WGDC 2022 Global Young Scientist Award, the Jack and Laura Dangermond Graduate Fellowship, UT MGCE Fellowship, 2025 Geospatial Rising Star Award, etc. He is currently the registration chair of ACM SIGSPATIAL 2025, vice chair of AAG GISS Specialty Group, and PC member for NeurIPS, ICML, ICLR, WWW, AISTATS, ACM SIGIR, ACM SIGSPATIAL, GIScience, etc.
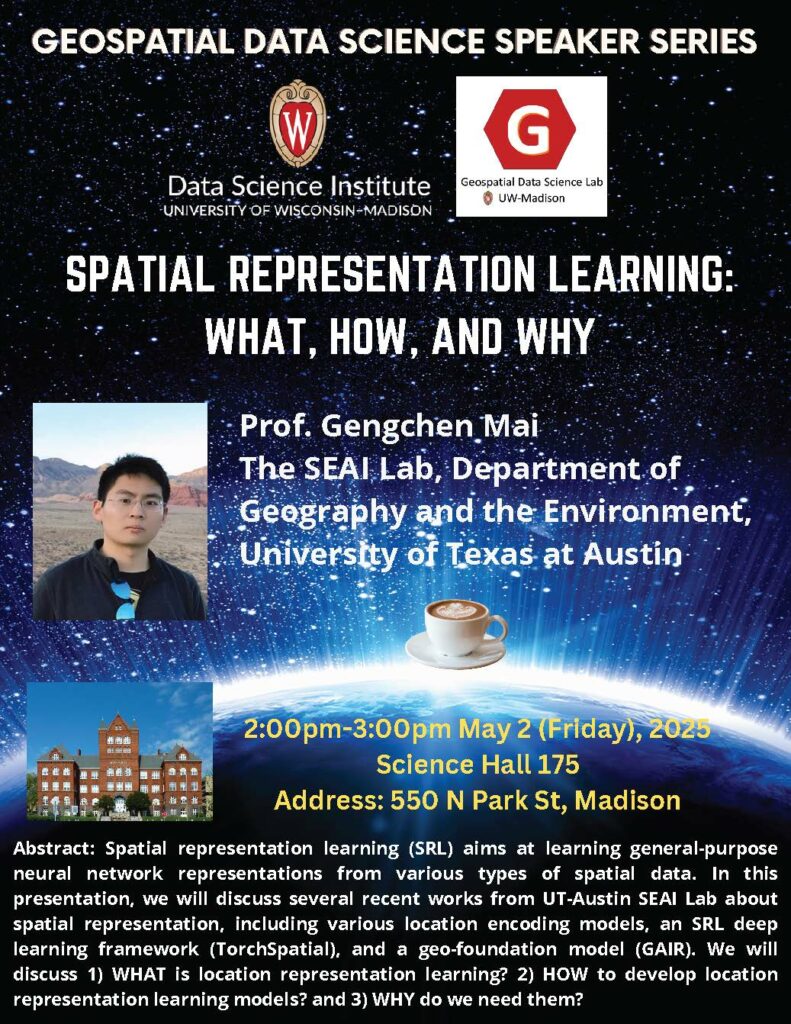