Yunlei Liang, Kyle W. McNair, Song Gao, Aslıgül Göçmen. (2021). Exploring Store Visit Changes During the COVID-19 Pandemic Using Mobile Phone Location Data. In Shih-Lung Shaw and Daniel Sui (Eds): Mapping COVID-19 in Space and Time: Understanding the Spatial and Temporal Dynamics of a Global Pandemic (Chapter 13). pp. 253-275, Springer.
Abstract:
When the World Health Organization (WHO) announced the pandemic of COVID-19, people around the globe scattered to stores for groceries, supplies, and other miscellaneous items in preparation for quarantine. The dynamics of retail visits changed dramatically due to the pandemic outbreak. The study intends to analyze how the store visit patterns have changed due to the lockdown policies during the COVID-19 pandemic. Using mobile phone location data, we build a time-aware Huff model to estimate and compare the visiting probability of different brands of stores over different time periods. We are able to identify certain retail and grocery stores that have more or fewer visits due to the pandemic outbreak, and we detect whether there are any trends in visiting certain retail establishments (e.g., department stores, grocery stores, fast-food restaurants, and cafes) and how the visiting patterns have adjusted with lockdowns. We also make comparisons among brands across three highly populated U.S. cities to identify potential regional variability. It has been found that people in large metropolitan areas with a well-developed transit system tend to show less sensitivity to long-distance visits. In addition, Target, which is a department store, is found to be more negatively affected by longer-distance trips than other grocery stores after the lockdown. The findings can be further applied to support policymaking related to public health, urban planning, transportation, and business in post-pandemic cities.
Highlighted results:
- The dwell time distribution of visitors in Target.
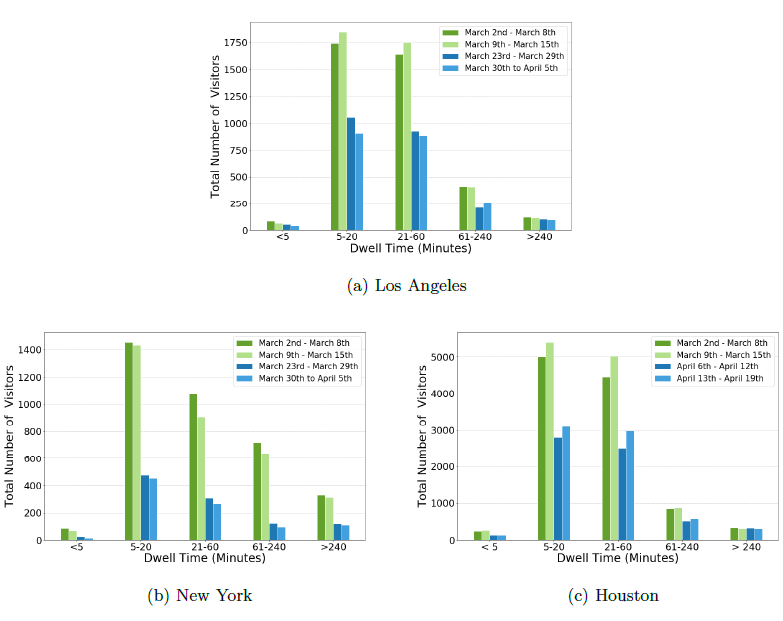
- Frequency of Visits from home Census Block Groups to Whole Foods Markets.
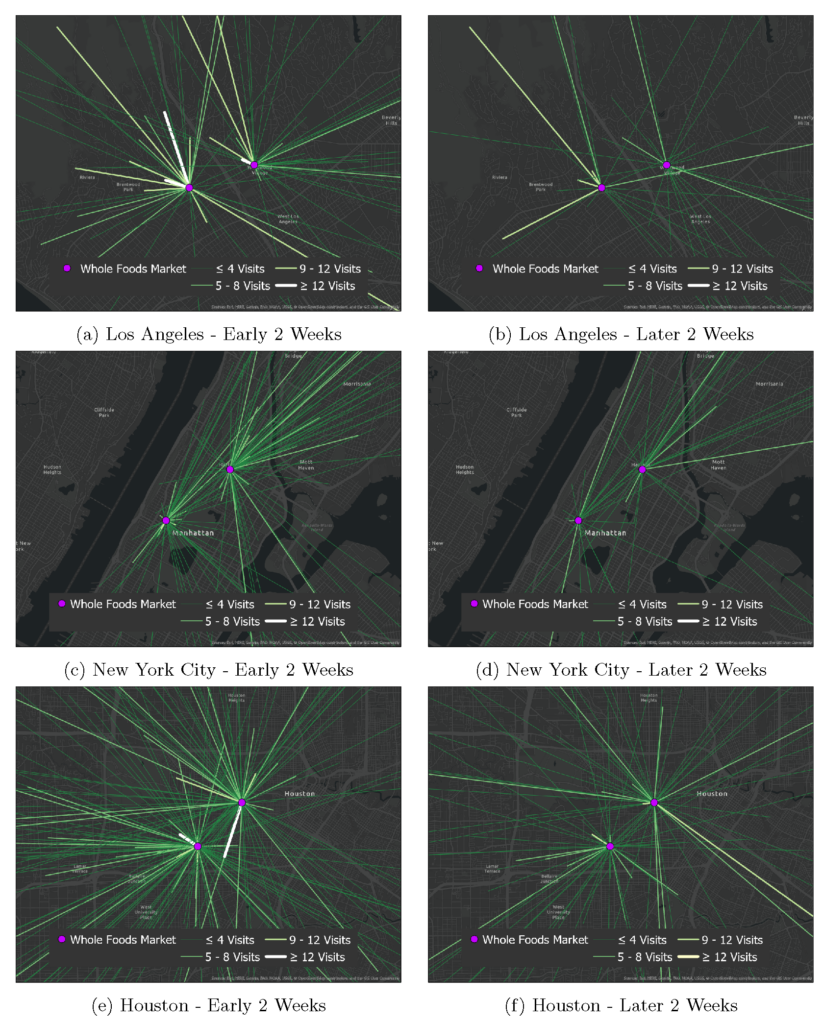