Research article published at the journal of Environment and Planning A: Economy and Space on December 1, 2019
Timothy Prestby, Joseph App, Yuhao Kang, Song Gao. (2019) Understanding Neighborhood Isolation through Spatial Interaction Network Analysis using Location Big Data. Environment and Planning A: Economy and Space. DOI: 10.1177/0308518X19891911
Download link: https://journals.sagepub.com/doi/full/10.1177/0308518X19891911
Abstract
Hidden biases of racial and socioeconomic preferences shape residential neighborhoods throughout the USA. Thereby, these preferences shape neighborhoods composed predominantly of a particular race or income class. However, the assessment of spatial extent and the degree of isolation outside the residential neighborhoods at large scale is challenging, which requires further investigation to understand and identify the magnitude and underlying geospatial processes. With the ubiquitous availability of location-based services, large-scale individual-level location data have been widely collected using numerous mobile phone applications and enable the study of neighborhood isolation at large scale. In this research, we analyze large-scale anonymized smartphone users’ mobility data in Milwaukee, Wisconsin, to understand neighborhood-to-neighborhood spatial interaction patterns of different racial classes. Several isolated neighborhoods are successfully identified through the mobility-based spatial interaction network analysis.
Keywords: Neighborhood isolation, human mobility, big data, spatial interaction
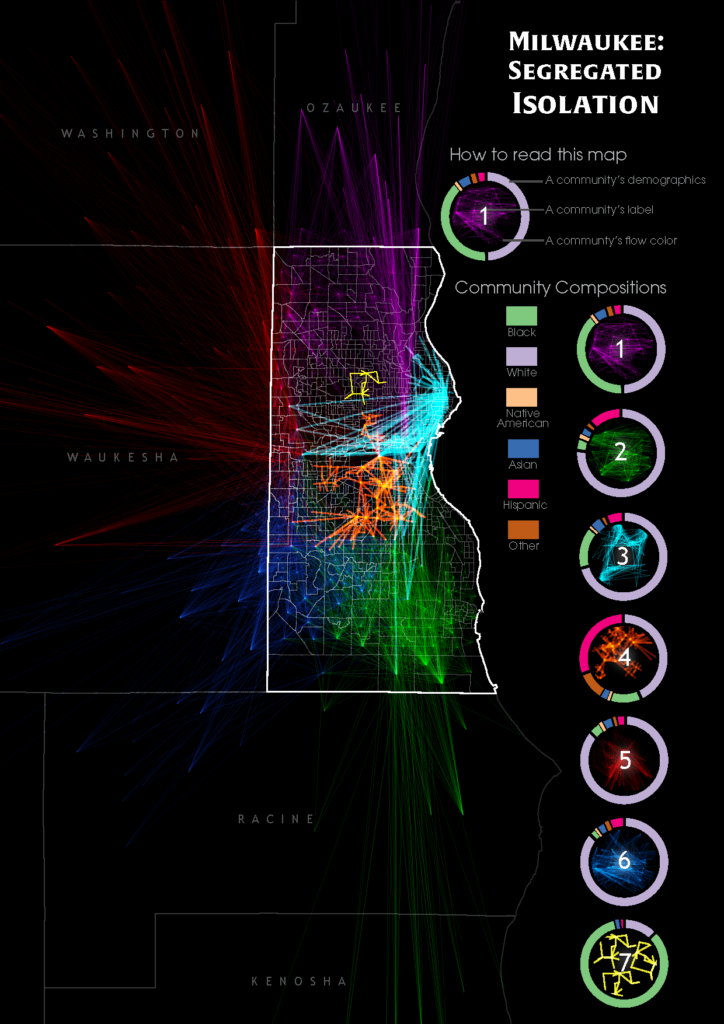
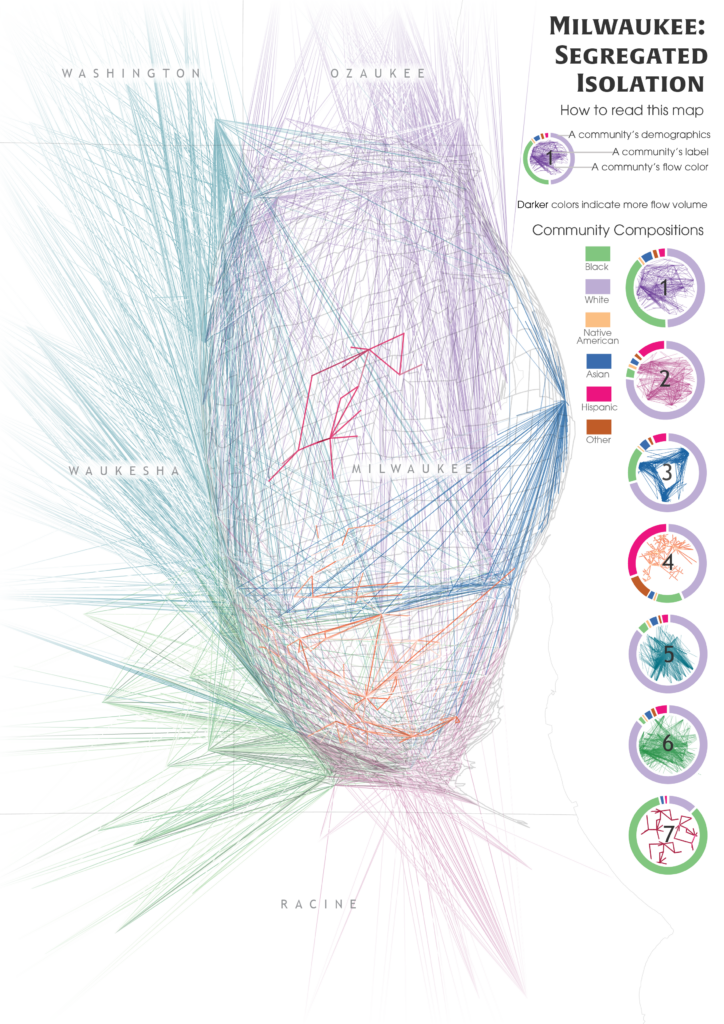
Acknowledgments
The authors would like to thank Safegraph, Inc., for providing the anonymous mobile phone location big data support. T.P. and S.G. thank the UW-Madison Hilldale Undergraduate/Faculty Research Fellowship for their support for this research.