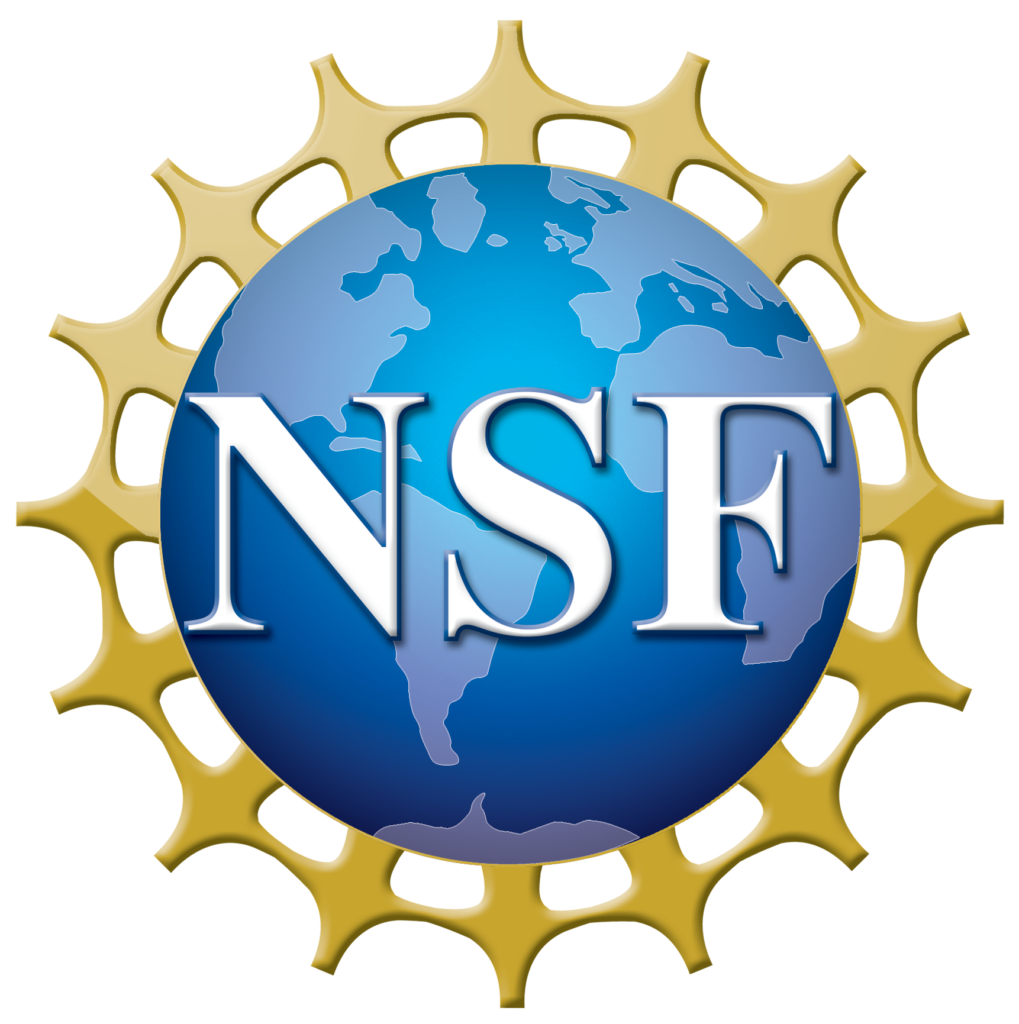
Recently, a multidisciplinary research team led by Prof. Song Gao (Geography) who serves as the Principal Investigator (PI) and collaborates with three other Co-PIs at UW-Madison: Prof. Kaiping Chen (Life Sciences Communication), Prof. Qin Li (Mathematics), and Prof. Jonathan Patz (Population Health Sciences), was awarded an NSF RAPID grant in response to the COVID-19 pandemic. The project title is: “Geospatial Modeling of COVID-19 Spread and Risk Communication by Integrating Human Mobility and Social Media Big Data”.
This project will investigate the gap between the science of epidemic modeling and risk communication to the general public in response to the COVID-19 pandemic. With the rapid development of information, communication, and technologies, new data acquisition and assessment methods are needed to evaluate the risk of epidemic transmission and geographic spreading from the community perspective, to help effectively monitor social distancing policies, and to understand social disparities and environmental contexts in risk communication. This project will make theoretical, methodological, and practical contributions that advance the understanding of the COVID-19 spread across both time and space. The communication aspects of this research will serve to educate communities about the science, timing, and geography of virus transmission in order to enhance actions for addressing such global health challenges. This project explores the capabilities and potential of integrating social media big data and geospatial artificial intelligence (GeoAI) technologies to enable and transform spatial epidemiology research and risk communication. Results will be disseminated broadly to multiple stakeholder groups. Further, this project will support both researchers and students from underrepresented groups, broadening participation in STEM fields. Lastly, the Web platform developed in this project will serve as an education tool for students in geography, communication, mathematics, and public health, as well as for effectively engaging with communities about the science of COVID-19.
Past health research mainly focuses on quantitative modeling of human transmission using various epidemic models. How to effectively communicate the science of an epidemic outbreak to the general public remains a challenge. When an epidemic outbreak occurs without specific controls in place, it can be particularly challenging to improve community risk awareness and action. The research team, composed of experts from geography, mathematics, public health and life sciences communication will (1) develop innovative mathematical predictive models that integrate spatio-temporal-social network information and community-centered approaches; (2) integrate census statistics, human mobility and social media big data, as well as policy controls to conduct data-synthesis-driven and epidemiology-guided risk analysis; And (3) utilize panel surveys and text mining techniques on social media data for better understanding public awareness of COVID-19 and for investigating various instant message and visual image strategies to effectively communicate about risks to the public. The results of this project will lead to a better understanding of the geography and spread of COVID-19. Additionally, it is expected that the methods developed in this project can be applied to mitigate the outbreak risks of future epidemics.
The research team will also collaborate with The Wisconsin State Cartographer’s Office (SCO), The Wisconsin Department of Health Services (DHS), The American Family Insurance Data Science Institute (DSI), and The Global Health Institute (GHI).
Read our recent work: Mobile location big data can help predict the potential infected areas as coronavirus spreads