During the week of November 1-4, 2022, all the GeoDS lab members were traveling to two academic conferences: ACM SIGSPATIAL 2022 and AutoCarto 2022.
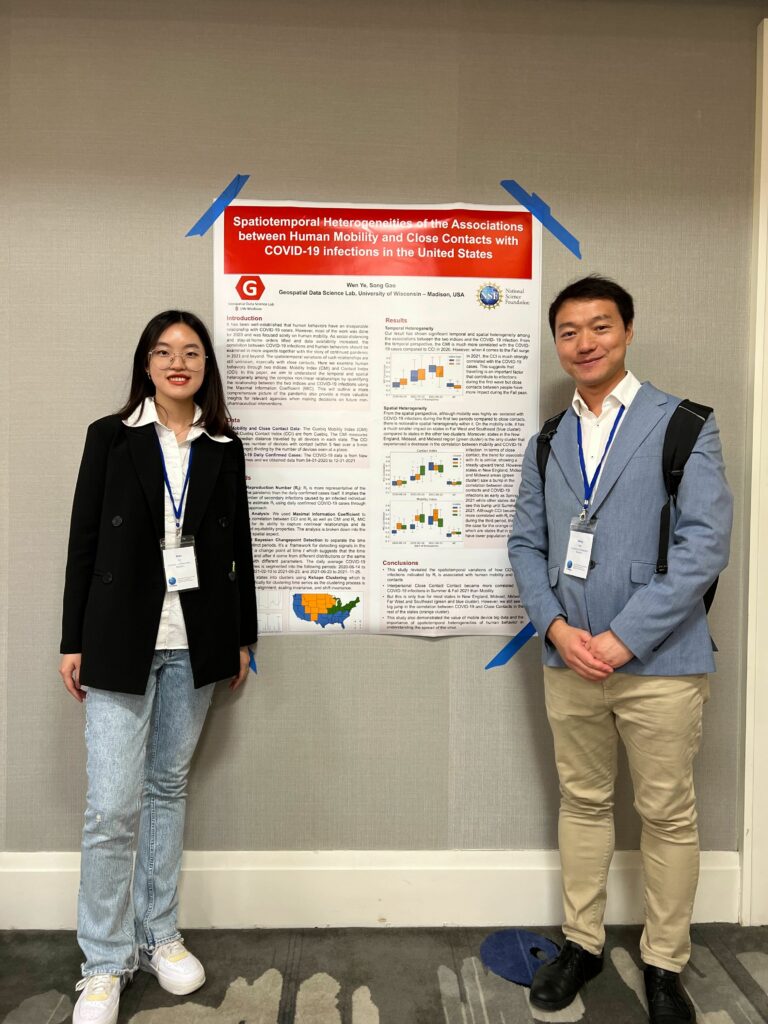
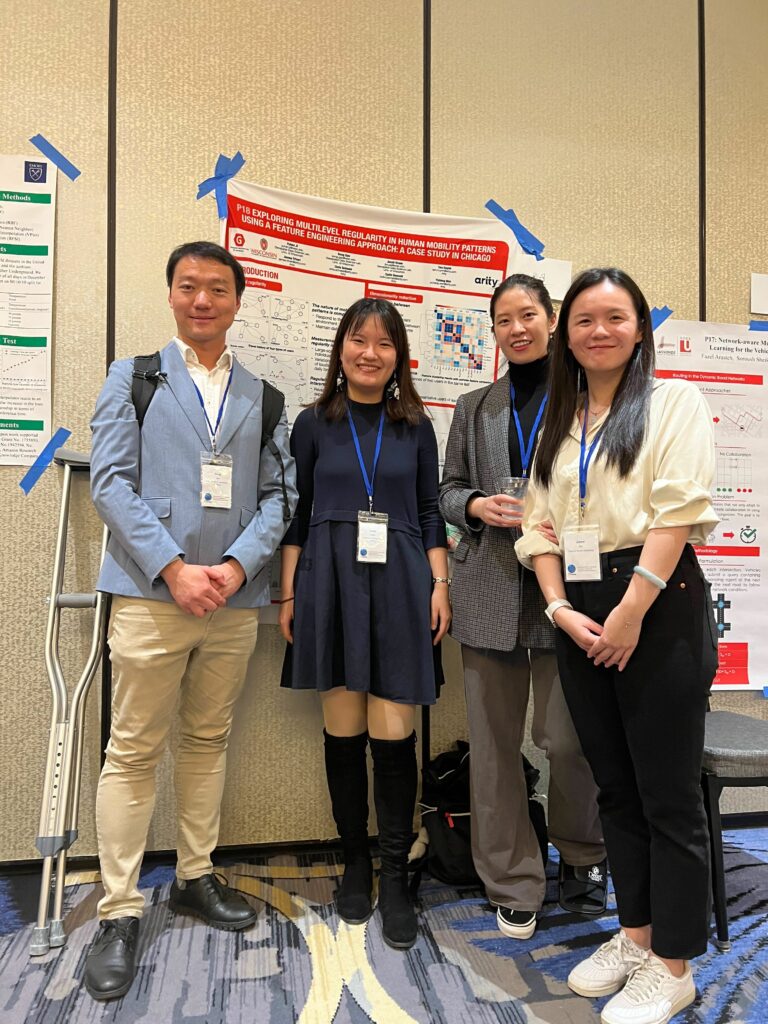
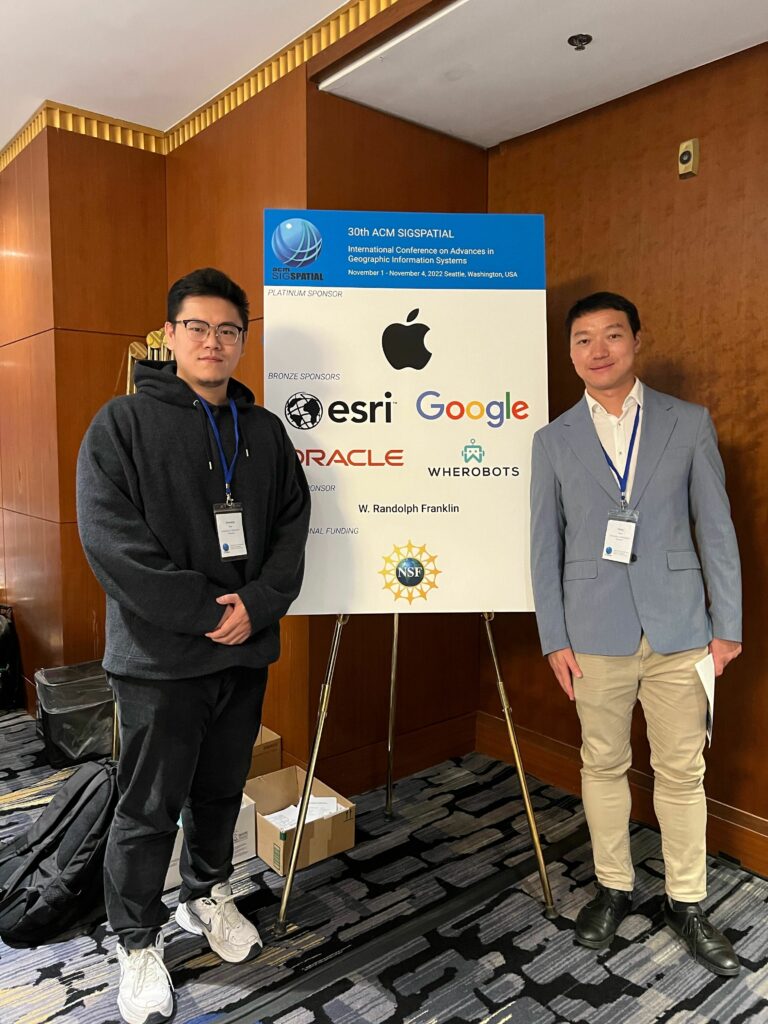
Prof. Song Gao, Wen Ye (undergraduate student), Yunlei Liang (PhD student), Yuhan Ji (PhD student), Jiawei Zhu (visiting PhD student), and Jinmeng Rao (PhD Candidate), presented at the 30th ACM SIGSPATIAL International Conference on Advances in Geographic Information Systems (ACM SIGSPATIAL 2022) in Seattle, Washington, USA.
We published two short research papers in the main conference, three workshop full papers, and won a “Best Paper Award”.
- Region2Vec: Community Detection on Spatial Networks Using Graph Embedding with Node Attributes and Spatial Interactions. Yunlei Liang, Jiawei Zhu, Wen Ye, Song Gao. (2022) In SIGSPATIAL’22, DOI:10.1145/3557915.3560974
- Exploring multilevel regularity in human mobility patterns using a feature engineering approach: A case study in Chicago. Yuhan Ji, Song Gao, Jacob Kruse, Tam Huynh, James Triveri, Chris Scheele, Collin Bennett, and Yichen Wen. (2022) In SIGSPATIAL’22, DOI:10.1145/3557915.3560974
- (Best Paper Award) Understanding the spatiotemporal heterogeneities in the associations between COVID-19 infections and both human mobility and close contacts in the United States. Wen Ye, Song Gao. (2022) In SpatialEpi ’22, pp 1–9, DOI:10.1145/3557995.3566117
- Measuring network resilience via geospatial knowledge graph: a case study of the us multi-commodity flow network. Jinmeng Rao, Song Gao, Michelle Miller, Alfonso Morales. (2022) In GeoKG’22, pp 17-25, DOI:10.1145/3557990.3567569
- Towards the intelligent era of spatial analysis and modeling. Di Zhu, Song Gao, Guofeng Cao. (2022) In GeoAI’22, pp 10-13, DOI:10.1145/3557918.3565863
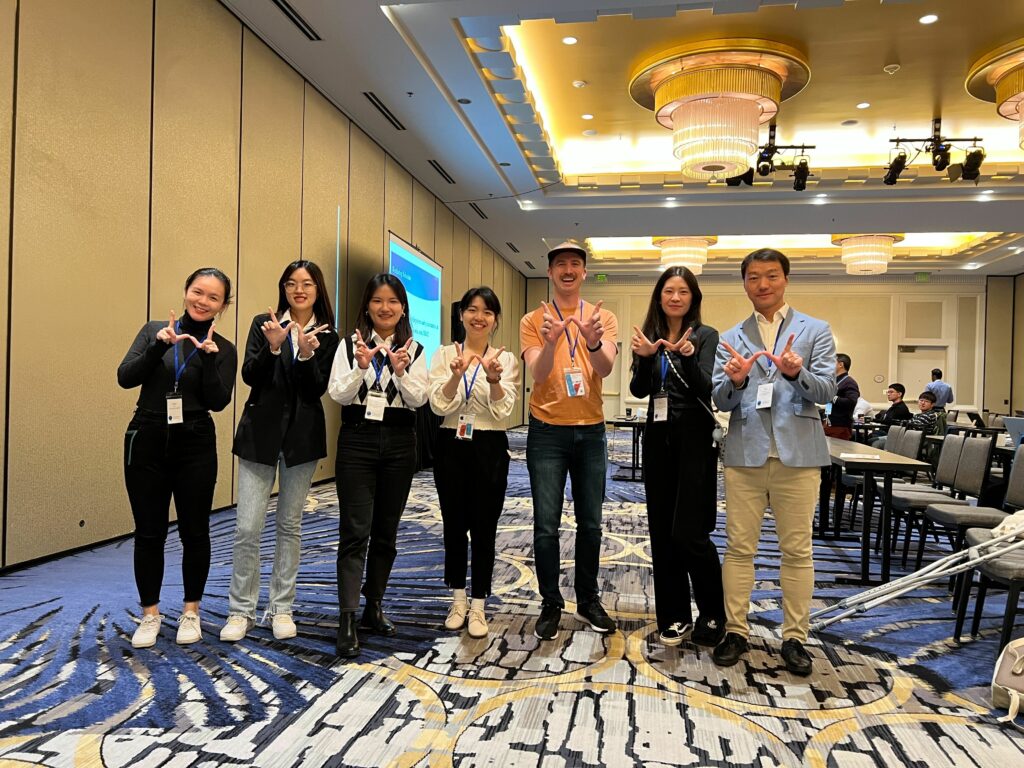
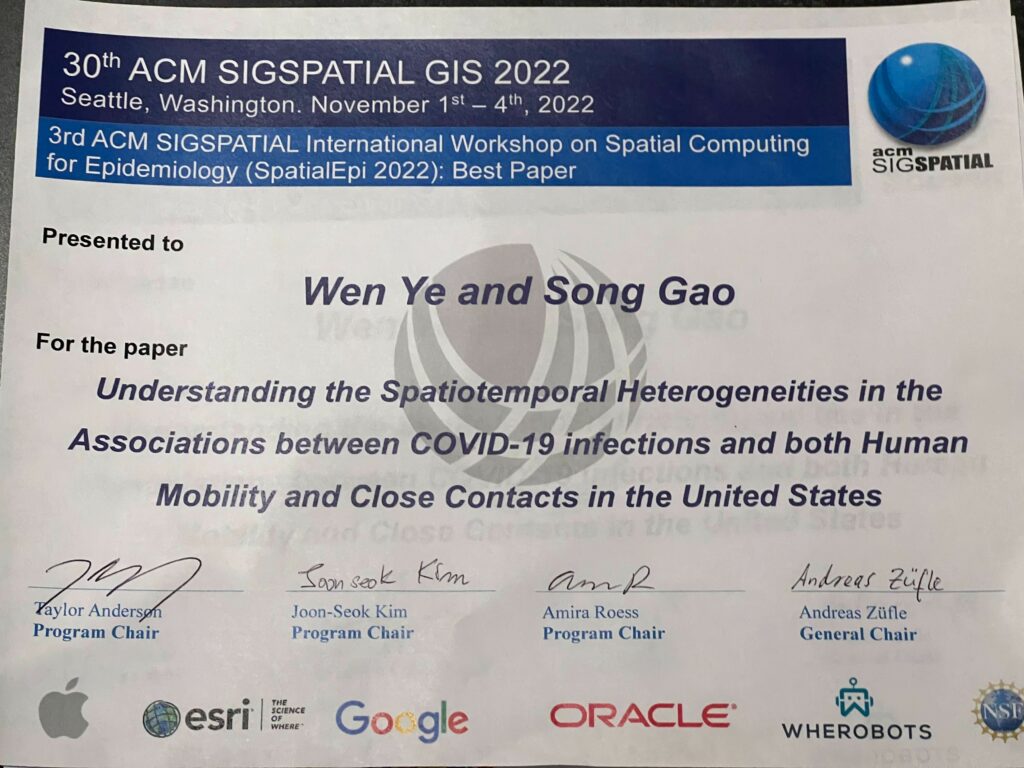
As the Proceedings Chairs, Professor Song Gao co-organized the 5th ACM SIGSPATIAL International Workshop on AI for Geographic Knowledge Discovery (GeoAI’22). There are two keynotes from both industry and academia and 12 oral presentations in the GeoAI workshop. The proceedings of the GeoAI’22 workshop is available at the ACM Digital Library: https://dl.acm.org/doi/proceedings/10.1145/3557918
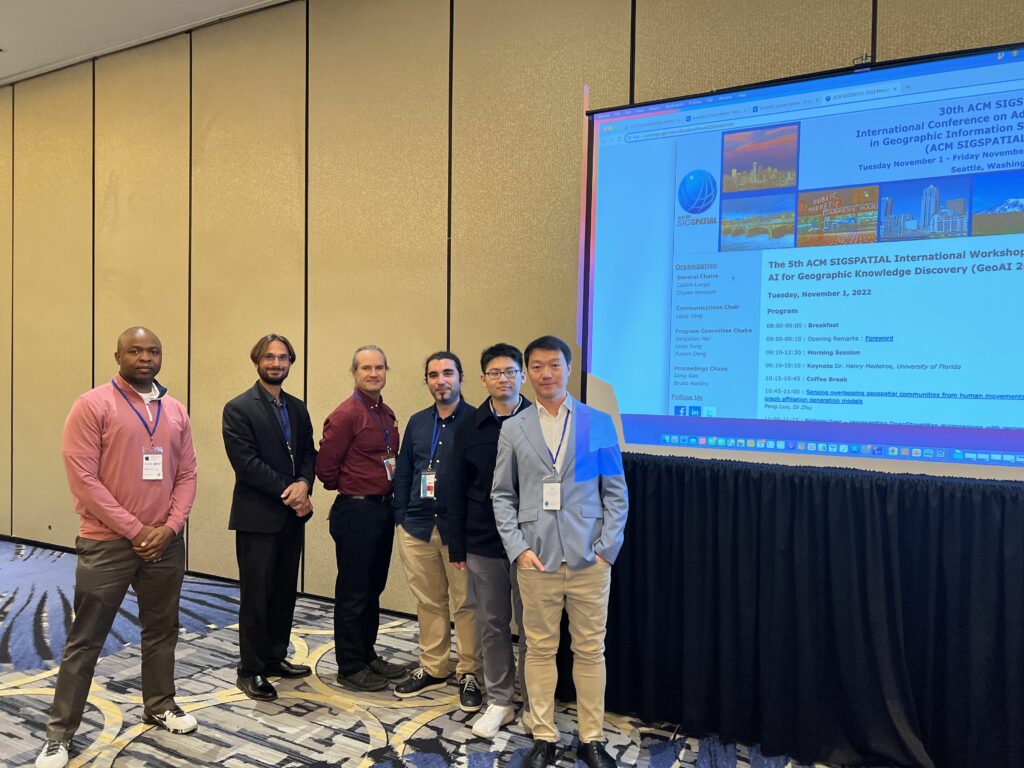
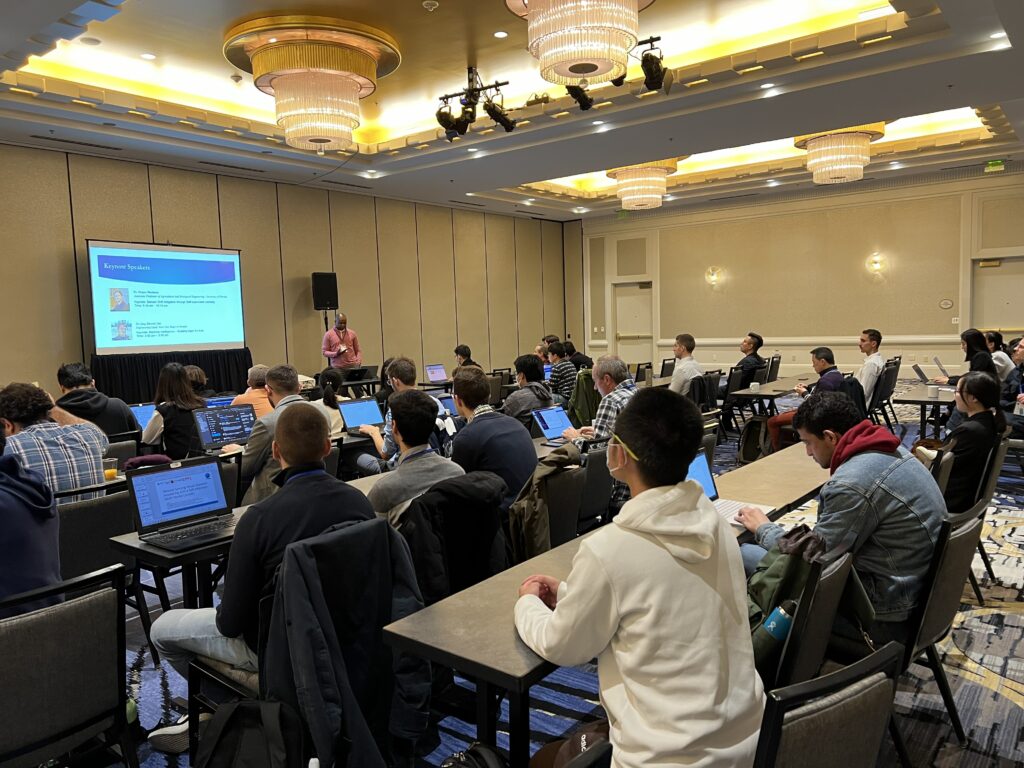
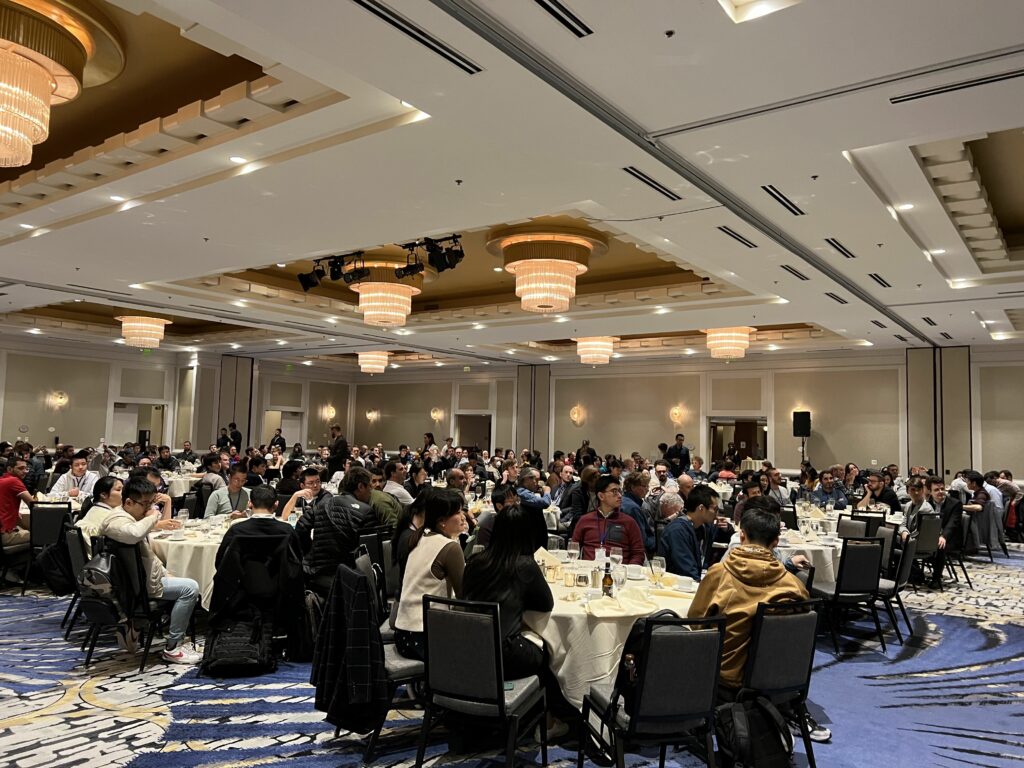
In addition, Yuhao Kang (PhD Candidate) and Jake Kruse (PhD Student) presented two short papers in the AutoCarto 2022, the 24th International Research Symposium on Cartography and GIScience.
- A Review and Synthesis of Recent GeoAI Research for Cartography: Methods, Applications, and Ethics. Yuhao Kang, Song Gao, Robert Roth (2022)
- Interactive Web Mapping for Multi-Criteria Assessment of Redistricting Plans. Jacob Kruse, Song Gao, Yuhan Ji and Kenneth Mayer (2022)
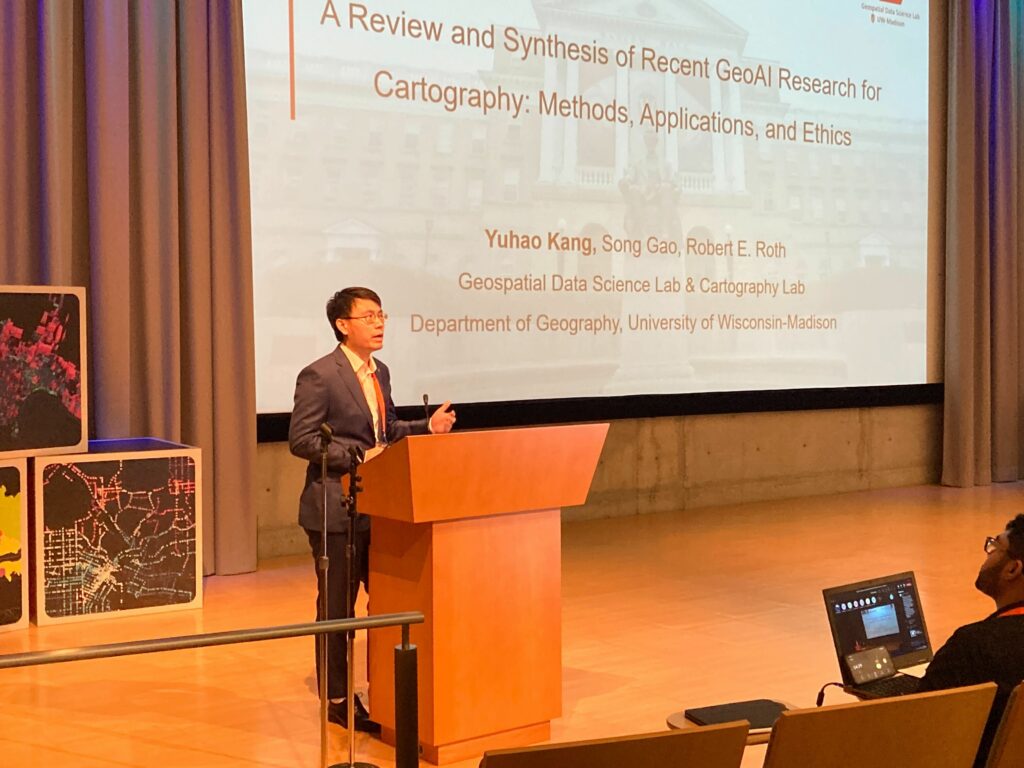
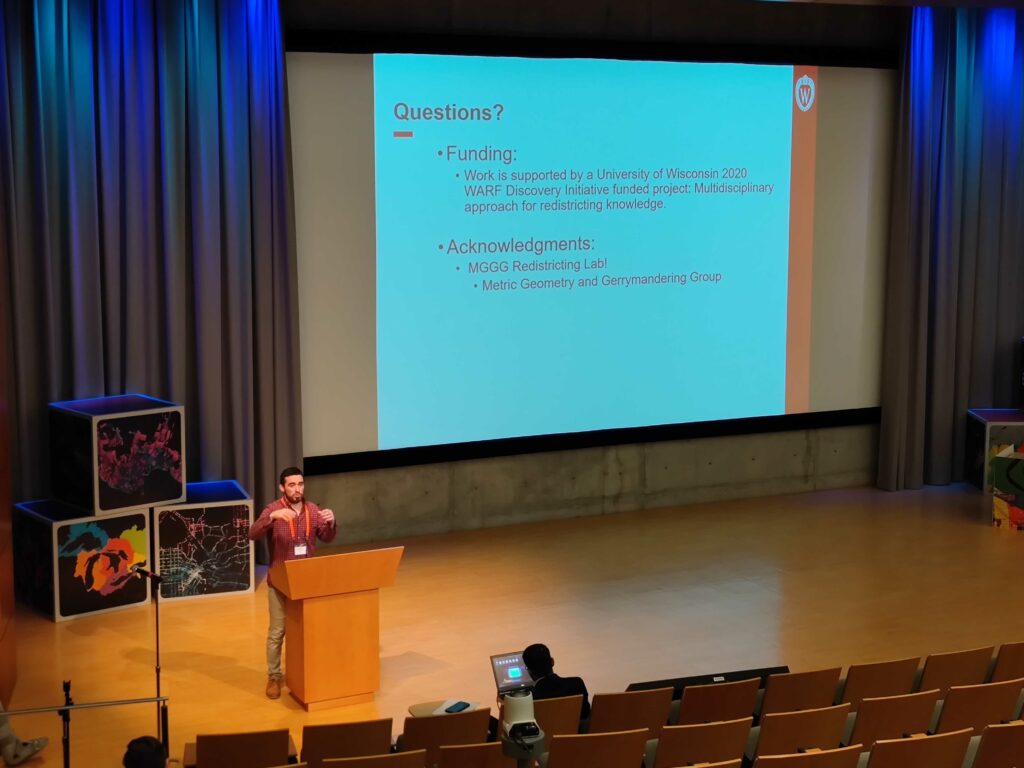
Also, Congrats to Yuhao who won the International Cartographic Association (ICA) Scholarship Award!
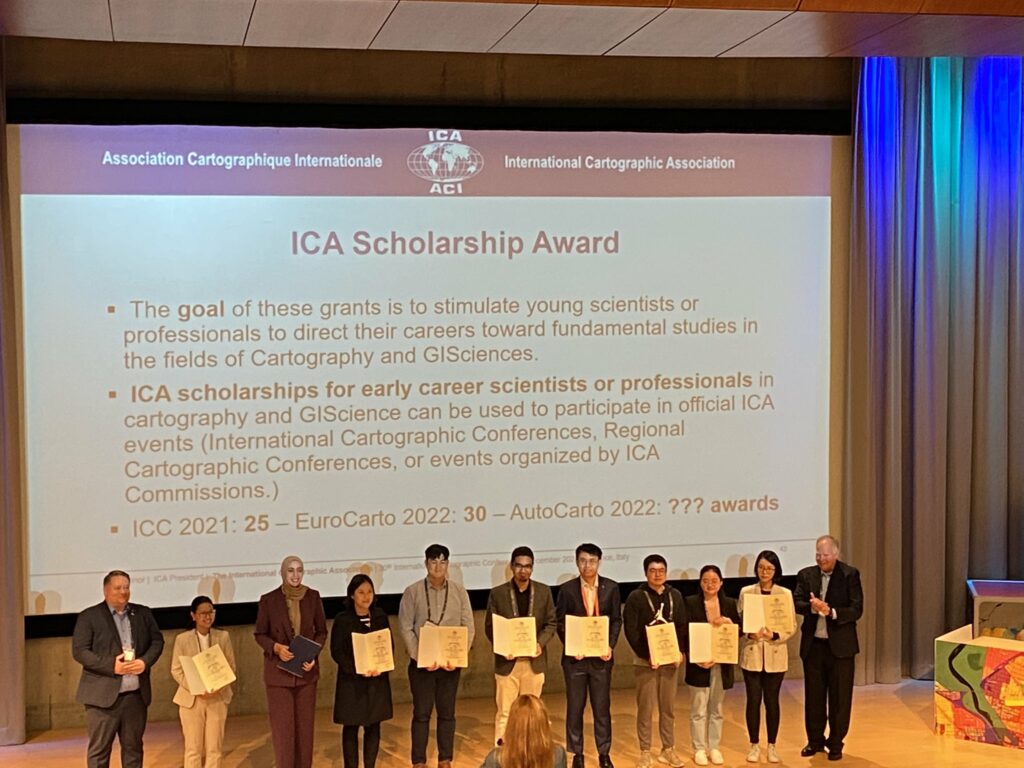